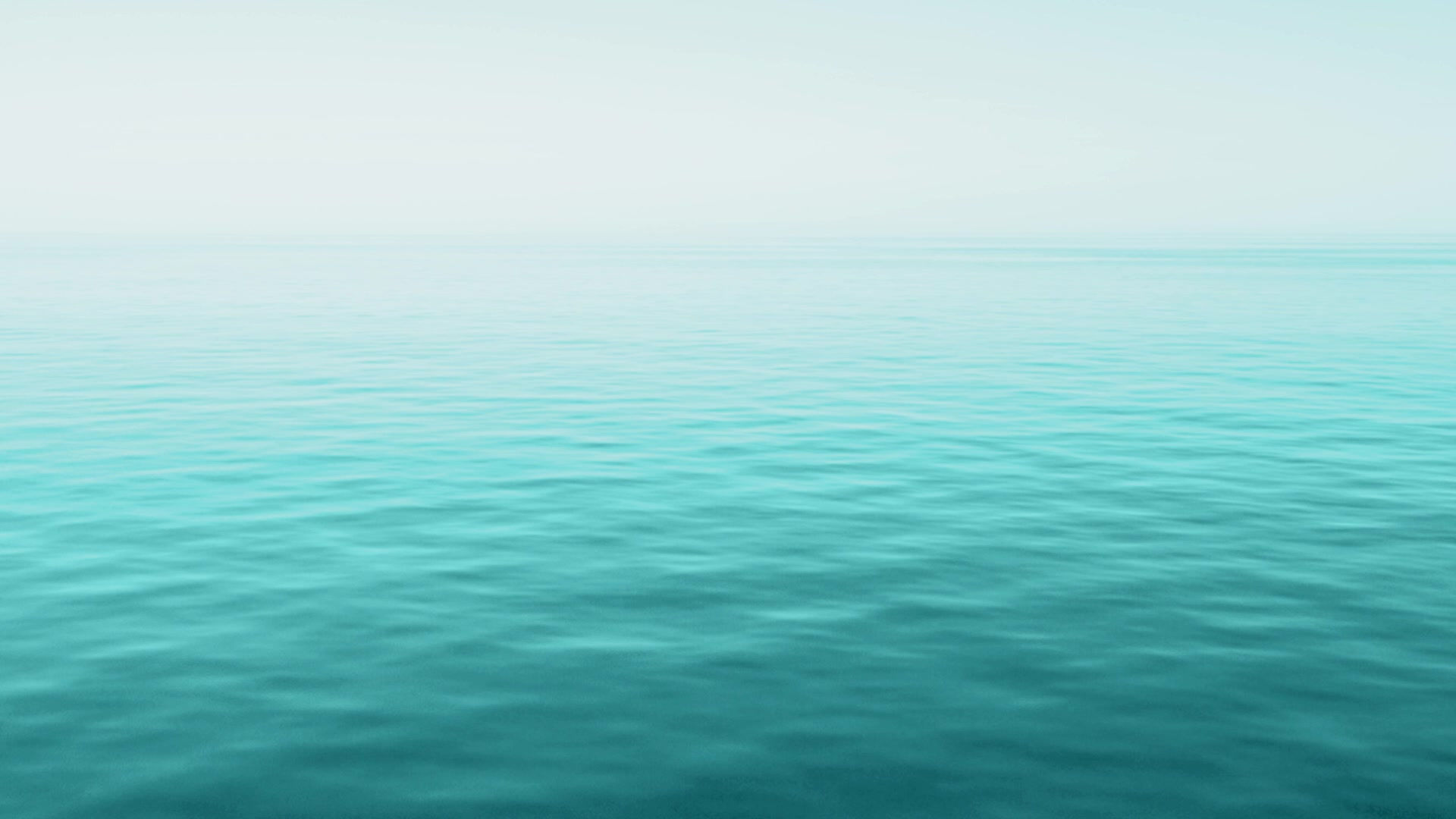
Suman Bhattacharyya
Latest Articles
Article | 03
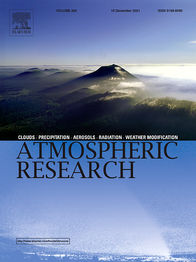
Characteristics of extreme rainfall in different gridded datasets over India during 1983–2015
S Bhattacharyya, S Sreekesh, A King
DOI: 10.1016/j.atmosres.2021.105930
Abstract
Understanding the spatial and temporal occurrences of extreme rainfall events and their changes is crucial for reducing the risk associated with extreme events, especially under anthropogenic warming. This study presents a comparative analysis of twelve gridded rainfall datasets in representing the spatial and temporal variation of extreme rainfall events and trends in the recent past (1983–2015) in India. The selected datasets fall into four categories: gauge-based (APHRODITE, CPC, GPCC, REGEN), satellite-derived (CHIRPS, PERSIANN-CDR), reanalysis (ERA5, MERRA-2, PGF, and JRA-55), and product merged from these three types (WFDEI and MSWEP). For comparison, a gauge-based, high resolution (0.25° × 0.25°) daily gridded rainfall dataset, prepared by the India Meteorological Department (IMD) is used as a reference dataset. Eleven extreme climate indices, defined by the World Meteorological Organization's Expert Team on Climate Change Detection and Indices (ETCCDI) and the IMD are used to represent the magnitude, frequency, and duration of extreme rainfall events in India. The relative performance of these datasets in capturing extreme rainfall events is evaluated by computing model evaluation matrices i.e. correlation coefficient (CC) and Percentage Bias (PBIAS). Trends in extreme rainfall indices and their magnitudes are calculated using Mann-Kendell and Sen's slope methods respectively, to compare with the IMD dataset. Our study finds large uncertainties in representing extreme rainfall events where in most cases the datasets underestimate higher extreme events compared to the IMD data. GPCC and MSWEP better capture the magnitude, duration, and frequency of extreme rainfall events in India and are comparable to the pattern of the IMD dataset. CHIRPS and ERA5 perform better than other satellite and reanalysis rainfall datasets. All these selected datasets consistently underestimate extreme rainfall events over the northern Himalayan region. The gridded datasets are unable to capture the spatial pattern of observed trends in extreme rainfall indices in India when compared to the IMD dataset.
Article | 02
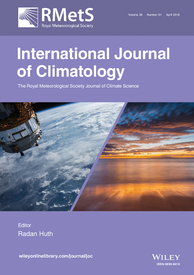